Introduction
With the rapid advancement of artificial intelligence (AI), its application in the field of mental health is gaining increasing attention. AI in mental health care leverages technologies such as emotion recognition, natural language processing (NLP), and predictive analytics to assist in identifying potential mental health conditions. AI-powered tools can provide emotional regulation support, psychological interventions, and even personalised therapy. Large language models (LLMs), such as GPT-based conversational systems, have demonstrated the ability to simulate active listening and empathy, helping users manage stress and emotional distress.
However, the use of AI in mental health care is not without challenges. AI lacks deep emotional understanding, making it difficult to provide truly individualised interventions. Ethical and privacy concerns also arise, particularly regarding data security and the risks associated with relying on AI for psychological well-being. This article explores the key applications, potential benefits, and inherent risks of AI in mental health care, aiming to provide a comprehensive understanding of its role and future prospects in digital healthcare.
Definition
Mental Health
Mental health refers to an individual’s emotional, psychological, and social well-being, influencing how they think, feel, and behave. It also affects how people handle stress, relate to others, and make decisions. Good mental health is essential for overall well-being and is a critical factor in maintaining a balanced life. Mental health disorders, including depression, anxiety, and schizophrenia, impact millions of people worldwide. According to the World Health Organisation (WHO), nearly one in four people globally will experience a mental health condition at some point in their lives (WHO). Furthermore, data from the World Mental Health (WMH) surveys across 29 countries estimates that by age 75, nearly one in two individuals will develop at least one mental disorder (McGrath et al., 2023). This highlights the urgent need for effective treatment and early intervention to mitigate symptoms and enhance long-term mental well-being.
AI in Mental Health Care
AI in healthcare refers to the use of machine learning, deep learning, NLP, and predictive analytics to improve medical diagnosis, treatment, and patient management. AI-powered tools analyse large datasets, recognise patterns, and provide evidence-based recommendations to healthcare professionals. In mental health, AI applications range from chatbots for psychological support, emotion recognition tools, and AI-driven diagnostics to personalised treatment recommendations. AI enhances the efficiency of healthcare delivery, enabling earlier intervention, continuous patient monitoring, and improved accessibility to mental health services. However, challenges such as bias in AI models, ethical concerns, and lack of emotional intelligence highlight the importance of responsible AI implementation in mental health care. For example, AI models trained predominantly on Western populations may misinterpret emotional expressions in individuals from diverse cultural backgrounds, leading to inaccurate assessments. Similarly, gender biases in training data can result in the underdiagnosis of certain mental health conditions in women or overdiagnosis in men. Addressing these biases is crucial to ensuring fair and effective AI-driven mental health solutions.
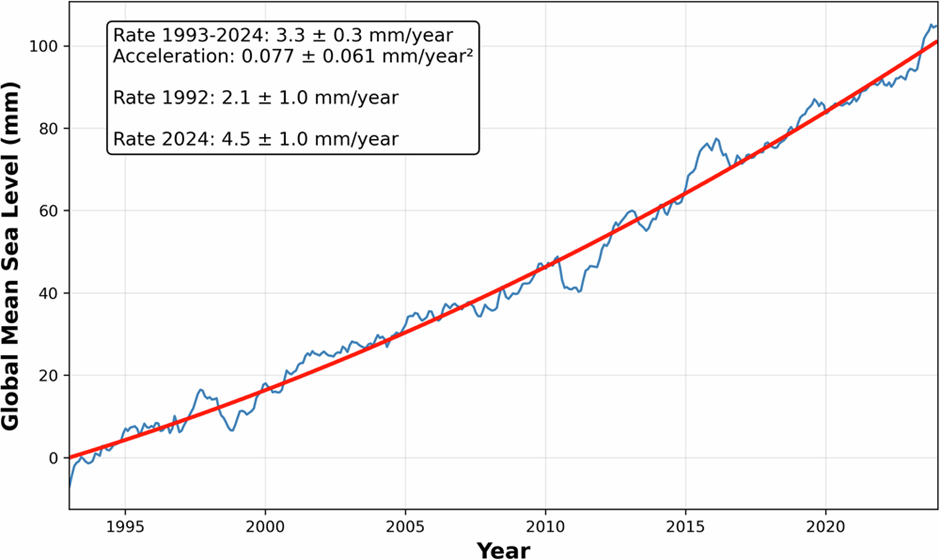
Source: Catherine K Ettman, Sandro Galea
applications of AI in Mental Health care
AI-Powered Chatbots and Virtual Therapists
AI-driven chatbots, such as Woebot, Wysa, and Replika, provide cognitive behavioural therapy (CBT) techniques, mindfulness exercises, and 24/7 emotional support. These virtual assistants allow individuals to manage stress, anxiety, and depression without waiting for in-person consultations (Haque & Rubya, 2023).
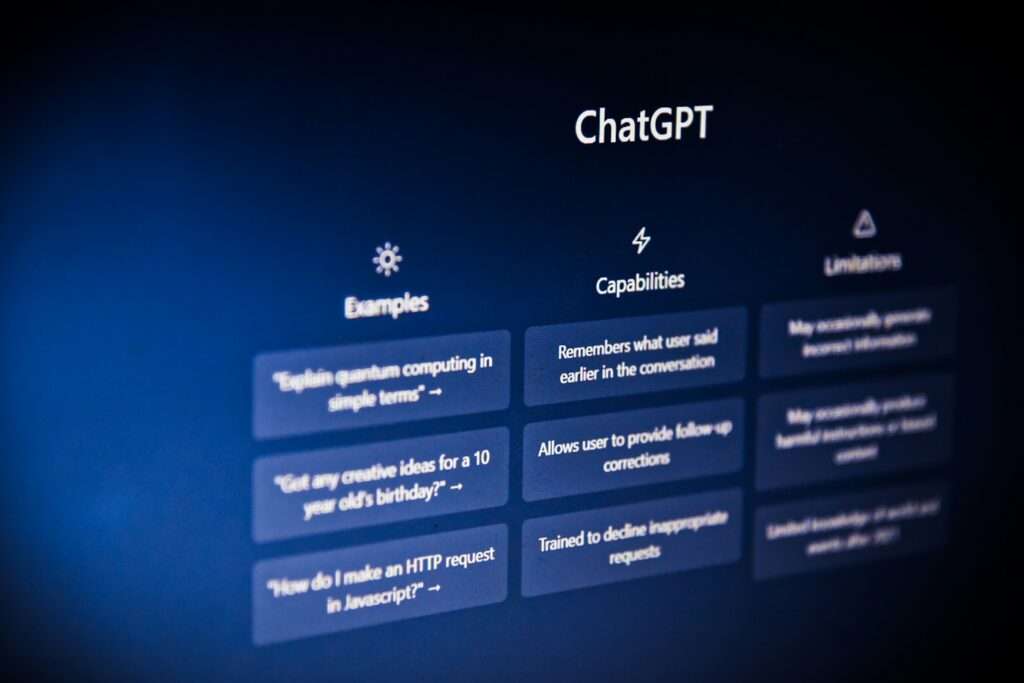
Source: Unsplash
AI in Diagnosing Mental Health Conditions
AI models utilise speech recognition, facial expression analysis, and NLP(Nature Language Processing) to detect early signs of depression, anxiety, bipolar disorder, and schizophrenia. Research indicates that AI-based diagnostic tools can analyse voice tone, word choice, and social media activity to predict mental health conditions with up to 90% accuracy (Kalmady et al., 2023).
Emotion Recognition and Sentiment Analysis
AI-driven emotion recognition tools analyse facial expressions, vocal tone, and text-based communications to assess emotional states in real time. These tools are used in digital therapy applications and online counselling to enhance patient monitoring. AI integrated with video conferencing platforms can detect non-verbal cues and suggest adjustments in therapeutic approaches for clinicians. Nandwani and Verma (2021) explain that Recurrent Neural Networks (RNNs), particularly Long Short-Term Memory (LSTM) models, are widely used in emotion recognition and sentiment analysis owing to their ability to capture long-term dependencies in text data.
Issues and Limitations of AI in Mental Health Care
While AI in mental health care presents significant opportunities for early issue detection, personalised interventions, and scalable mental health support, it also faces several critical limitations and challenges. These issues stem from technological constraints, ethical concerns, and the need for human oversight, all of which must be addressed for AI to function as a reliable tool in mental health services.
The Challenge of Emotional Intelligence Deficiency
One of the primary challenges is the lack of deep emotional intelligence in AI models. While AI-driven chatbots and virtual assistants can simulate empathy using sentiment analysis and NLP, they lack the ability to truly understand and respond to human emotions with genuine sensitivity. AI systems struggle with nuance, cultural variations in emotional expression, and the unpredictability of human emotions, which can lead to misinterpretations or inadequate responses, particularly in high-risk situations (Oritsegbemi, 2023). For example, an AI chatbot may fail to recognise suicidal intent in a distressed user owing to indirect language. If the system misinterprets urgency, it might provide generic advice instead of escalating the case for immediate human intervention. Such errors can delay critical support and worsen mental health crises.
Algorithmic Bias and Inequality in AI Models
Another major concern is bias in AI algorithms. AI models learn from historical datasets, which may contain racial, gender, and socioeconomic biases that can negatively impact mental health diagnoses and treatment recommendations. Research has shown that algorithmic bias in AI-driven diagnostics can lead to disparities in mental health care, particularly for marginalised communities (Huang et al., 2024). If AI systems are trained on limited or non-representative data, they risk reinforcing existing inequalities rather than improving accessibility. Addressing this requires diverse, high-quality datasets and continuous bias auditing in AI model development.
Privacy, Security, and Ethical Concerns
Additionally, privacy and ethical concerns remain significant obstacles to AI adoption in mental health. AI-based mental health tools often collect highly sensitive personal data, raising concerns about data security, informed consent, and potential misuse. A breach of confidential mental health records could lead to stigmatisation, discrimination, or even legal consequences for patients. Regulatory frameworks such as the General Data Protection Regulation (GDPR) and the Health Insurance Portability and Accountability Act (HIPAA) provide guidelines for data protection, but there is still a lack of standardised global policies governing the ethical use of AI in digital mental health (Morley, 2022).
Over-Reliance on AI and Psychological Risks
Another critical limitation is the risk of over-reliance on AI in mental health care. Mucci and Marazziti (2023) highlight that anxiety disorders may experience heightened symptoms owing to AI-driven decision-making processes, where patients might develop excessive dependence on algorithmic recommendations, reinforcing uncertainty and distress when faced with ambiguous situations. Similarly, individuals with Obsessive-Compulsive Disorder (OCD) could be negatively affected by the predictability and precision of AI responses, exacerbating compulsive behaviours and rigid thought patterns as they seek AI-validated certainty. Furthermore, for individuals with Attention Deficit Hyperactivity Disorder (ADHD), AI interactions may provide constant digital stimulation, making it harder to sustain focus in offline environments and potentially increasing distractibility in daily life.
Future use of AI in mental Health care
The future of AI in mental health care will transform how conditions are monitored, diagnosed, and treated. As AI technology evolves, its integration with neuroscience and wearable health devices will improve mental healthcare. Human-AI collaboration models will enable more proactive, personalised, and scalable mental health solutions.
One key future direction is AI-driven neurotechnology for improving mental health diagnostics and interventions. Brain-computer interfaces (BCIs) combined with AI will enable real-time brain activity monitoring. This will offer insights into conditions like depression, schizophrenia, and Post-traumatic Stress Disorder (PTSD). Also, these advancements will enhance the detecting accuracy and prediction of cognitive and emotional states. Clinicians can tailor treatment plans using neurological biomarkers instead of relying only on self-reported symptoms. AI-assisted neuromodulation therapies, like transcranial magnetic stimulation (TMS) and deep brain stimulation (DBS), will become more precise and personalised. These innovations will improve outcomes for individuals with treatment-resistant mental health disorders (Luca Cocchi, 2024).
Another promising area is AI-enabled digital twins for mental health patients. A digital twin is a virtual model of an individual’s psychological and physiological state. It updates continuously using real-time data from wearable sensors, genetic information, and behavioural assessments. This technology enables highly customised mental health interventions, adjusting treatment plans dynamically as a patient’s condition evolves. AI-powered digital twins simulate and predict treatment responses, helping clinicians make data-driven decisions. It minimises trial-and-error in psychiatric care, improving treatment precision and patient outcomes.
Conclusion and Call to Action (CTA)
AI is transforming mental health care by diagnosing, treating, and monitoring disorders. AI-driven technologies, including predictive analytics and digital twins, enhance accessibility and improve treatment precision. Neurotechnology and immersive therapy expand mental health support beyond clinical settings, providing innovative solutions.
However, AI models face challenges, including bias, data privacy concerns, and the risk of over-reliance on automated systems. Addressing these issues is crucial to ensuring AI remains an ethical and effective tool in mental health care.
To maximise AI’s benefits, stakeholders—including clinicians, researchers, developers, policymakers, and advocates—must collaborate on transparent ethical guidelines. Improving AI interpretability and integrating AI solutions with human-centred mental health services will enhance its effectiveness.
Mental health professionals should use AI as a supplement rather than a replacement, ensuring AI-driven interventions support therapeutic relationships. Governments and healthcare organisations must invest in regulatory frameworks that ensure data security, algorithmic fairness, and patient autonomy.
Continued research and public awareness initiatives can bridge the gap between AI innovation and clinical applications. Fostering trust in AI-assisted mental healthcare solutions is essential for widespread adoption.
Achieving the UN Sustainable Development Goals (SDGs)
Integrating AI in mental health care aligns with the United Nations’ Sustainable Development Goals (SDGs) by improving access to mental health services, reducing inequalities, and fostering well-being on a global scale. SDG3: Good Health and Well-Being emphasises the importance of mental health as a fundamental aspect of overall health. AI-driven mental health tools provide early detection, personalised interventions, and scalable mental health support, making psychological care more accessible and efficient. SDG10: Reduced Inequalities is also supported by AI’s ability to bridge gaps in mental healthcare accessibility, particularly for underserved populations, individuals in remote areas, and those facing financial barriers.
A Thrivable Framework
Beyond sustainability, the concept of thrivability emphasises holistic and regenerative solutions that enhance human and societal well-being rather than simply mitigating harm. Applying the Thrive Framework to AI in mental health care means moving beyond reactive treatment models. It focuses on designing AI-powered systems. It will promote long-term resilience, self-awareness, and emotional well-being.
Ethical Values-Based Innovation should drive AI development, emphasising inclusivity, patient empowerment, and user-centred care over profit-driven models. To ensure long-term trust and effectiveness, AI must be designed for Strong Sustainability, avoiding short-term fixes or unsustainable data practices that compromise user safety and ethical governance.
A Regenerative Economic approach is essential to keeping AI-driven mental health tools affordable and accessible. This helps reduce rather than widen global mental health disparities. A Multi-Capital Approach must guide AI evaluation by measuring impact beyond financial terms. Social, emotional, and human capital must be considered to ensure well-being, equity, and resilience. Mental health solutions should enhance these aspects rather than focusing solely on efficiency.
At THRIVE, we are committed to redefining the future of mental healthcare by championing thrivability through AI innovation. Explore how AI in mental health care is transforming accessibility and personalised support by reading our in-depth research articles, expert insights, and ethical AI discussions. Stay informed by subscribing to our updates and newsletters, and join our interactive webinars where leading professionals discuss the evolving role of AI in mental well-being. Dive deeper with our podcast and blog series to uncover cutting-edge strategies and solutions that are shaping a thrivable, inclusive, and human-centred mental health landscape.